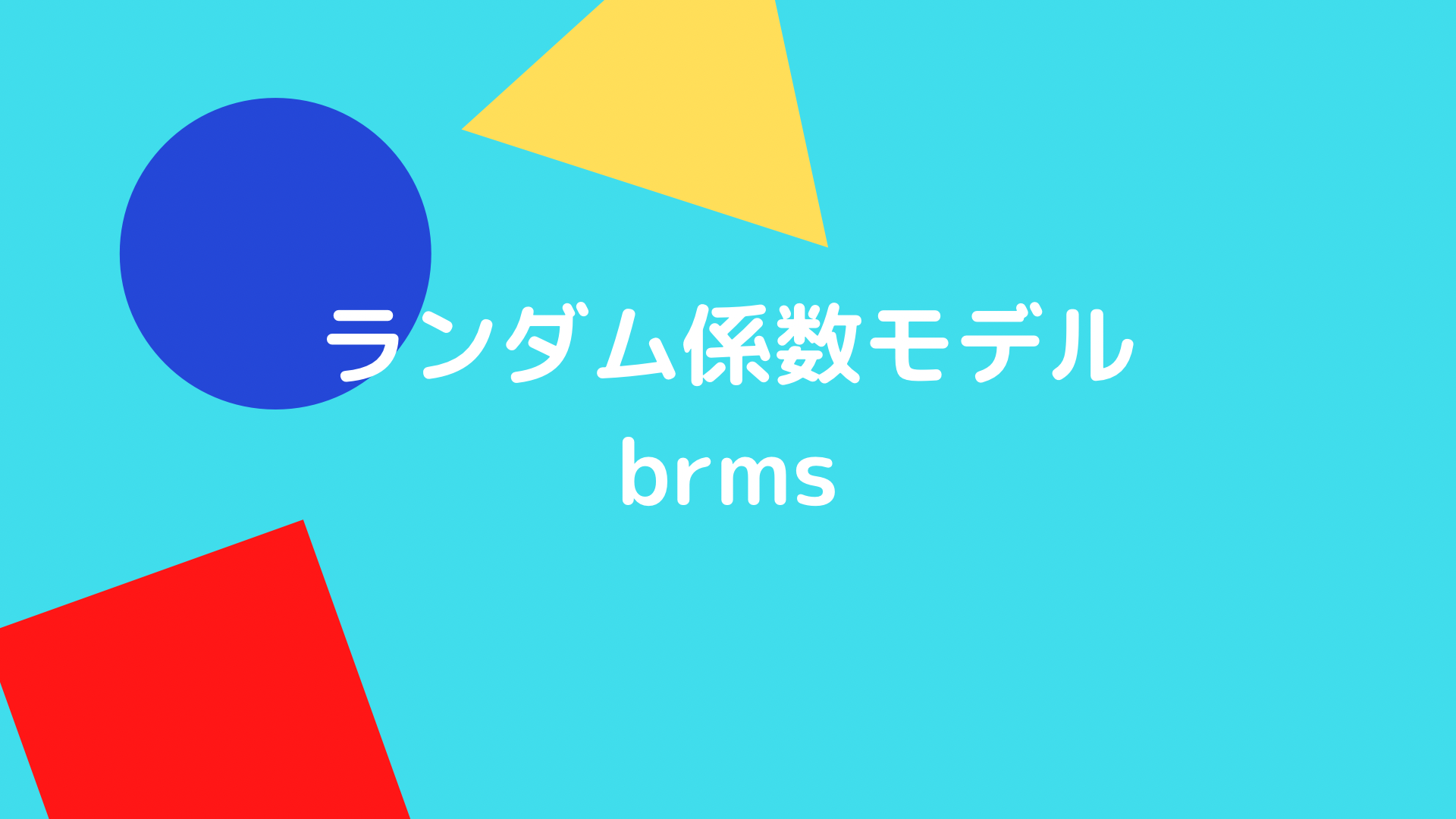
【Rでベイズ統計モデリング#15】ランダム係数モデル(brms)
記事の目的
GLMであるランダム係数モデルのベイズ推定を、RとStanを使用して実装していきます。今回は、「brms」というライブラリを使用します。データの作成から実装するので、コピペで再現することが可能です。
目次
0 前準備
0.1 今回のモデル
0.2 ワーキングディレクトリの設定
以下の画像のようにワーキングディレクトリを設定します。設定したディレクトリに、RファイルとStanファイルを保存します。
1 ライブラリ
# 1 ライブラリ library(dplyr) library(ggplot2) library(rstan) library(brms) library(patchwork) set.seed(1) rstan_options(auto_write=TRUE) options(mc.cores=parallel::detectCores())
2 データ
2.1 コード
# 2 データ 気温 <- rnorm(100,20,5) %>% round(1) 休日 <- rbinom(100, 1, 2/7) 店舗 <- runif(100, 1, 4) %>% round() r1 <- rnorm(4, 0, 0.6) r2 <- rnorm(4, 0, 0.4) data <- data.frame(気温, 休日, 店舗) %>% mutate(r1=ifelse(店舗==1, r1[1], ifelse(店舗==2, r1[2], ifelse(店舗==3, r1[3],r1[4]))))%>% mutate(r2=ifelse(店舗==1, r2[1], ifelse(店舗==2, r2[2], ifelse(店舗==3, r2[3],r2[4]))))%>% mutate(lambda=exp(-2+0.2*気温+(0.5+r1)*休日+r2)) %>% mutate(休日=factor(休日)) data$売り上げ個数 <- rpois(100, data$lambda) data %>% select(気温, 休日, 店舗, 売り上げ個数) %>% head() plot <- data %>% ggplot(aes(x=気温, y=売り上げ個数, color=休日)) + geom_point() + theme_classic(base_family = "HiraKakuPro-W3") + theme(text=element_text(size=25))+ labs(x="気温", y="売り上げ個数", title="データ") + facet_wrap(.~ 店舗) plot
2.2 結果
13行目の結果 | 22行目の結果 |
![]() |
![]() |
3 brmsの利用
# 3 brmsの使用 mcmc_result <- brm( data = data, formula = 売り上げ個数~ 気温 + 休日 + (休日||店舗), family = poisson(), seed = 1, iter = 2000, warmup = 200, chains = 4, thin=1 )
4 分析結果
4.1 コード
# 4 分析結果 ## 4.1 推定結果 print(mcmc_result) ranef(mcmc_result) ## 4.2 収束の確認 theme_set(theme_classic(base_size = 10, base_family = "HiraKakuProN-W3")) plot(mcmc_result) ## 4.3 λの確認 condition <- data.frame(店舗=1:4) plot(conditional_effects(mcmc_result, effects="気温:休日",re_formula=NULL, conditions = condition), points=TRUE, ncol=2) %>% wrap_plots() + plot_annotation(title="λの推定結果") ## 4.4 予測分布 plot(conditional_effects(mcmc_result, effects="気温:休日",re_formula=NULL, conditions = condition, method="predict"), points=TRUE, ncol=2)%>% wrap_plots() + plot_annotation(title="予測分布")
4.2 結果
3行目の結果 | 8行目の結果 |
![]() |
![]() |
12行目の結果 | 17行目の結果 |
![]() |
![]() |